Correlation and Causation Examples in Health
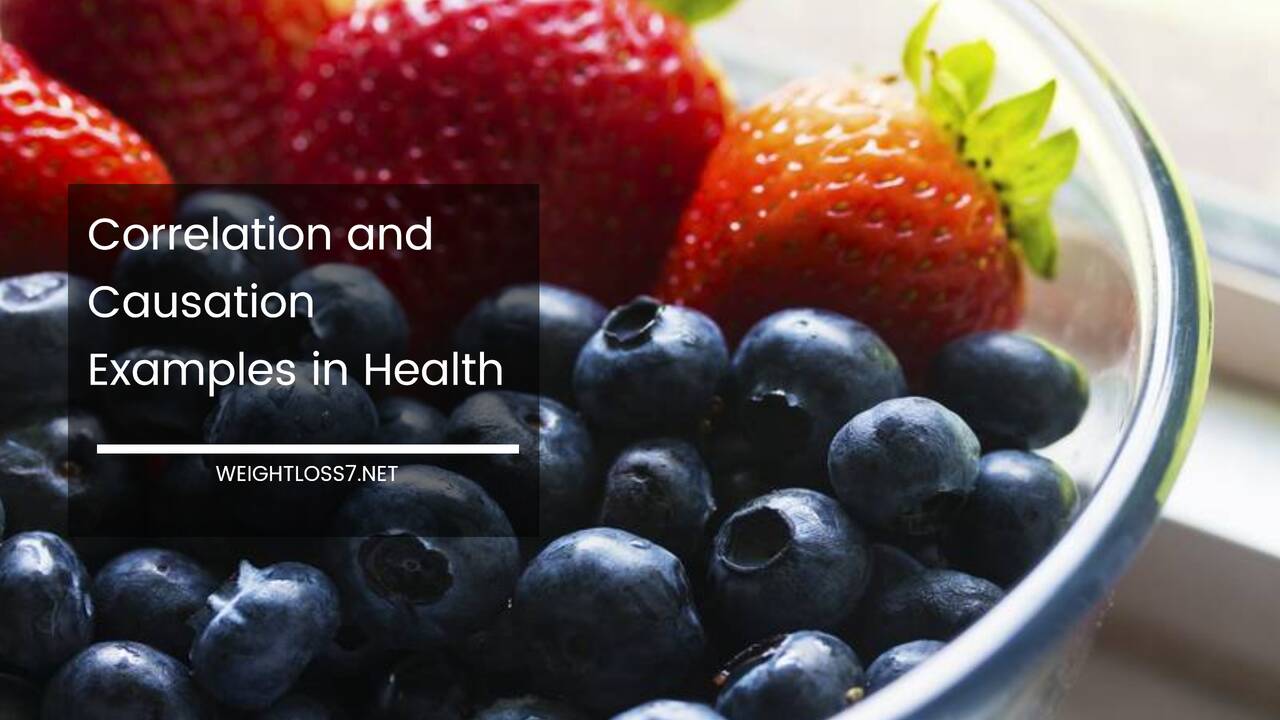
Correlation and Causation
Correlation and Causation Examples in Health: Unveiling the “Why” Behind the “What”
In the ever-evolving landscape of health research, headlines often bombard us with connections between seemingly disparate factors and health outcomes.
We’re bombarded with pronouncements like “Coffee linked to lower risk of dementia” or “Living near green spaces promotes mental well-being.”
These pronouncements can be enticing, prompting us to overhaul our morning routines or pack our bags for a nature retreat.
But before we dive headfirst into such lifestyle changes, it’s crucial to understand the fundamental distinction between correlation and causation.
Correlation vs. Causation: Decoding the Intricate Dance
Correlation simply describes a statistical relationship between two variables. Imagine a study revealing that people who drink coffee tend to live longer.
This is a correlation. However, causation implies that one variable directly influences the other. Here, we’d need compelling evidence demonstrating that coffee itself somehow extends lifespan. The difference might seem subtle, but the implications are vast.
The Pitfalls of Mistaking Correlation for Causation
Relying solely on correlations can lead us down a path of misconceptions. Consider these cautionary tales:
-
Ice Cream Sales and Drownings: Data might reveal a rise in ice cream sales alongside an increase in drownings. Does ice cream cause drowning? Absolutely not! Both factors likely correlate with a third, lurking variable: hot summer weather. People buy more ice cream and spend more time swimming when it’s hot, leading to a potential rise in drownings. This is a classic example of a confounding variable, a factor that influences both the independent and dependent variables in a study, skewing the results.
-
Education and Health: Studies often suggest that people with higher education levels tend to have better health outcomes. Does getting a degree guarantee good health? Unlikely. Perhaps socioeconomic factors influence both education and health access. Wealthier families might prioritize education and healthcare for their children, creating a correlation without a direct causal link. This scenario highlights the importance of considering socioeconomic status as a potential confounding variable in health research.
Unveiling Causation: The Scientific Sherlock Holmes
So, how do we move beyond correlation to establish causation? Here are some key strategies that scientists employ to play health research detective:
-
Randomized Controlled Trials (RCTs): Considered the gold standard for establishing causation, RCTs involve randomly assigning participants to groups. One group receives the “intervention” (e.g., a new drug, a specific dietary regimen), while the other receives a placebo (inactive substance) or a control condition. This helps isolate the impact of the intervention and minimize the influence of external factors. While RCTs are powerful tools, they can be expensive and time-consuming to conduct, and they may not be ethical or feasible for all types of research, particularly those involving long-term health outcomes.
-
Longitudinal Studies: These studies track participants over an extended period, allowing researchers to observe how changes in one variable might influence another over time. For example, a longitudinal study might follow a group of children from birth into adulthood, examining how their diet and lifestyle choices in childhood influence their health outcomes later in life. However, longitudinal studies can also be susceptible to confounding variables and may be limited by the challenges of maintaining participant engagement over long periods.
-
Biological Plausibility: Does the proposed causal link make biological sense? Understanding the underlying mechanisms strengthens the case for causation. For instance, research suggests that regular exercise might improve mental health by increasing levels of neurotransmitters like endorphins, which elevate mood. Here, scientists can investigate the biological pathways involved to bolster the evidence for causation.
Correlation in Health Research: Stepping Stones to Causation
While correlation doesn’t guarantee causation, it can act as a valuable springboard for further investigation. Here’s how correlations can serve as stepping stones to uncovering causal relationships:
-
Generating Hypotheses: Observed correlations can spark ideas for future research. The coffee-longevity link might prompt scientists to explore potential protective compounds in coffee that could explain its association with a longer lifespan. This can lead to the development of more targeted studies aimed at establishing causation.
-
Identifying High-Risk Groups: Correlations can help pinpoint populations with a higher likelihood of developing certain health problems. For example, a correlation between air pollution and respiratory illnesses might guide public health initiatives to target areas with high pollution levels, potentially leading to preventive measures.
-
Refining Research Questions: Correlations can help refine research questions and guide the design of future studies. If a study finds a correlation between social isolation and depression, researchers might design a follow-up study to explore the potential mechanisms at play (e.g., loneliness affecting sleep quality, which then impacts mood).
Examples of Correlation and Causation in Health: A Spectrum of Evidence
Let’s delve into some real-world health examples that illustrate the spectrum of evidence, from intriguing correlations that require further investigation to well-established causal links that have informed public health policy.
Correlation:
- Cell Phone Use and Brain Tumors: Some studies have shown a correlation between frequent cell phone use and an increased risk of brain tumors. However, the evidence for causation is currently weak. More research, including long-term studies with robust methodologies, is needed to understand the potential effects of cell phone radiation on brain health.
Emerging Causation:
- Diet and Gut Microbiome: A growing body of research suggests a correlation between gut microbiome composition and various health conditions, including obesity, inflammatory bowel disease, and even mental health. While the research is ongoing, scientists are actively exploring the mechanisms by which gut bacteria might influence these conditions. This is an exciting area of research with the potential to establish stronger causal links in the future.
Established Causation:
- Smoking and Lung Cancer: The link between smoking and lung cancer is one of the most compelling examples of established causation in health research. Decades of research, including large-scale epidemiological studies and biological evidence, have firmly established that smoking directly damages lung tissue and increases the risk of cancer. This understanding has led to significant public health campaigns aimed at reducing smoking rates.
Challenges and Considerations:
Understanding correlation and causation in health research is not always a straightforward process. Here are some additional challenges to consider:
-
Reverse Causation: In some cases, the direction of causality might be reversed. For example, people who are predisposed to a certain health condition might unknowingly engage in behaviors that appear to be risk factors for that condition. This can make it difficult to tease out cause and effect.
-
Dosage and Timing: The relationship between variables can be dose-dependent or time-dependent. For example, the impact of exercise on mental health might vary depending on the intensity and duration of exercise. Causality studies need to consider these factors to accurately assess the relationship between variables.
-
Genetic Predisposition: Individual genetic makeup can influence how susceptible someone is to certain health risks. This can complicate the interpretation of both correlation and causation studies.
The Takeaway: Cultivating a Critical Eye
The next time you encounter a health claim, remember the importance of discerning correlation from causation. Here are some questions you can ask yourself to become a more informed consumer of health information:
- What type of research supports the claim? Are there RCTs, longitudinal studies, or just observational studies?
- Are there alternative explanations for the observed connection? Could confounding variables be at play?
- Is there a plausible biological mechanism underlying the proposed causal link?
- What is the source of the information? Is it a reputable scientific journal or a website with a less rigorous editorial process?
By developing a healthy dose of skepticism and asking critical questions, you can make informed decisions about your health and avoid falling prey to misleading information.
Ultimately, a nuanced understanding of correlation and causation is essential for navigating the ever-evolving world of health research.
Correlation and Causation in Mental Health
The interplay between correlation and causation is particularly complex in the realm of mental health. Here’s why:
-
Subjectivity of Mental Health Measures: Mental health is often assessed through self-reported questionnaires or clinician observations, which can be subjective and susceptible to bias. This inherent subjectivity can complicate the interpretation of correlations observed in mental health research.
-
Comorbidity: Mental health conditions often co-occur, making it difficult to isolate the effects of a single factor. For example, someone experiencing anxiety might also struggle with sleep problems. It becomes challenging to determine if one condition causes the other, or if they both stem from a common underlying cause.
-
Environmental and Social Factors: Mental health is significantly influenced by environmental and social factors such as poverty, trauma, and social isolation. These factors can be difficult to control for in research studies, potentially influencing observed correlations.
Examples in Mental Health:
-
Social Media Use and Depression: Studies have shown a correlation between increased social media use and symptoms of depression. However, the direction of causality remains unclear. Does social media use contribute to depression, or do people experiencing depression seek solace in social media? More research is needed to tease out these possibilities.
-
Exercise and Anxiety: Numerous studies suggest a positive correlation between regular exercise and reduced anxiety symptoms. The mechanisms at play are likely multifaceted, involving stress reduction, improved sleep quality, and neurotransmitter changes. While causation is not definitively established, the evidence suggests exercise can be a valuable tool in managing anxiety.
Moving Forward in Mental Health Research:
Despite the challenges, researchers are actively developing more sophisticated methods to study mental health:
-
Genetic Studies: Examining genetic variations can help identify individuals predisposed to certain mental health conditions. This knowledge can inform research on potential causal pathways and personalized treatment approaches.
-
Neuroimaging: Technologies like fMRI and PET scans allow researchers to investigate brain activity associated with mental health disorders. This can provide insights into the biological underpinnings of these conditions and potentially strengthen causal links.
-
Big Data Analysis: Large-scale analysis of electronic health records and social media data can reveal hidden patterns and associations that might inform future research directions.
The Importance of a Holistic Approach:
Ultimately, understanding mental health requires acknowledging the interplay between biological, psychological, and social factors.
Correlation studies can serve as valuable starting points, but robust research designs that consider the complexity of mental health are essential for establishing causal relationships and developing effective interventions.
The Future of Health Research: Moving Beyond the Binary
While the distinction between correlation and causation remains a cornerstone of research design, the future of health research might involve a more nuanced approach.
Emerging fields like network analysis and systems biology aim to understand complex interactions between multiple variables, potentially revealing intricate causal pathways that go beyond simple one-to-one relationships.
In conclusion, understanding the difference between correlation and causation is crucial for interpreting health research and making informed decisions about your well-being.
By recognizing the limitations of correlation and appreciating the power of well-designed studies aimed at establishing causation, we can cultivate a healthier and more evidence-based approach to managing our health.
Final Word: A Lifelong Journey
The pursuit of understanding the “why” behind health outcomes is a continuous quest. By fostering a critical lens towards correlation and causation, we can navigate the ever-evolving landscape of health research.
Remember, this is a lifelong journey of learning and self-discovery. By arming yourself with knowledge and a healthy dose of skepticism, you can make informed decisions about your health and well-being.